High Performance Computing
for Energy Innovation
In partnership with industry, leveraging world-class computational resources
to advance the national energy agenda.
NOW OPEN
HPC4EI is now accepting applications
for Spring 2024 Solicitation focused
on topic areas for HPC4Mtls and HPC4Mfg.
START HERE
Funding Opportunity
Project Statistics to Date

11
Participating Labs

182
Projects Awarded

$55.9 million
Funds Invested

916 million
Computer Core Hours
How the HPC4EI Program Works

Program Basics and Cost Sharing
The program pays labs up to $300K for industry access to
HPC resources and expertise; industry pays at least 20%
of project costs (cash or in-kind).
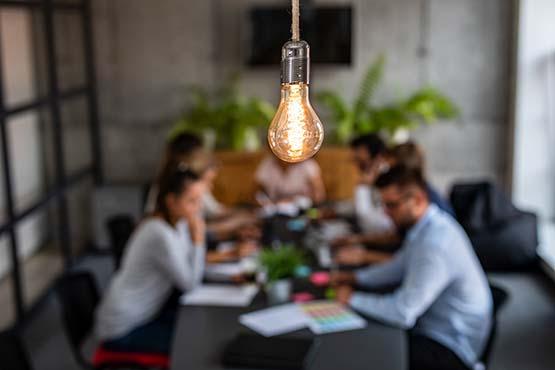
Concept Submissions
During a semiannual solicitation process, companies may
submit two-page concept papers describing ideas for projects
of up to one year duration.
Need more info about qualifications and submissions?
Check out our FAQs
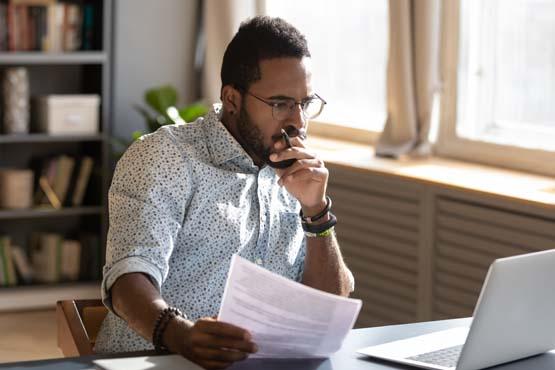
Lab Principal Investigator
If a concept is accepted, a DOE National Laboratory principal investigator is assigned to help the company develop a full proposal.
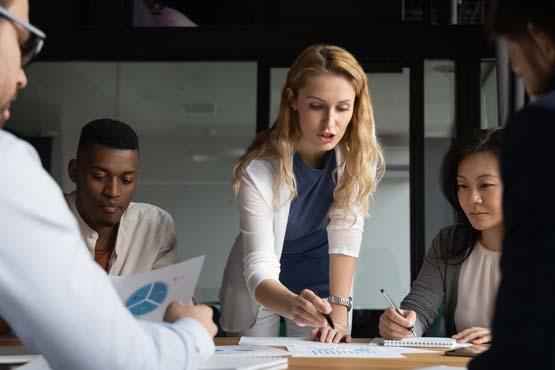
Selection Criteria
• Advances the State of the Art in the Industrial Sector
• Technical Feasibility
• Relevance to HPC
• Impact, including Life-cycle Energy Impact
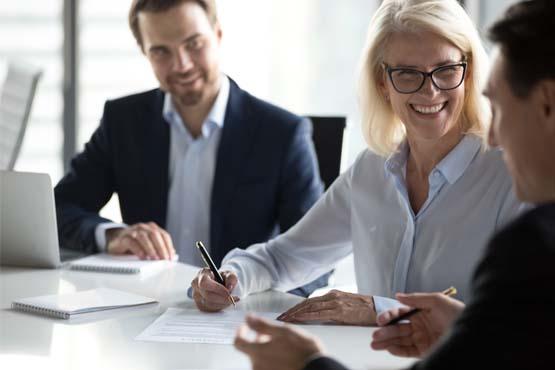
Signed Agreement
Following proposal approval, partnering DOE National Laboratory provides the company with a Short Form Cooperative Research and Development Agreement (CRADA) to initiate the project.
Need more information about the DOE Model Short Form CRADA?
Check out our FAQs
READY TO START?
Spring 2024 Solicitation
Accepting Applications
About HPC4EI
The High Performance Computing for Energy Innovation (HPC4EI) is the umbrella initiative for the High Performance Computing for Manufacturing (HPC4Mfg) Program and High Performance Computing for Materials (HPC4Mtls) Program.
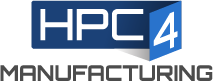
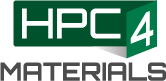
Through support from the Office of Energy Efficiency and Renewable Energy’s (EERE) Advanced Materials and Manufacturing Technologies Office (AMMTO), Industrial Efficiency and Decarbonization Office (IEDO), and the Office of Fossil Energy Carbon Management (FECM), the selected industry partners are granted access to high performance computing (HPC) facilities and world-class scientists at Department of Energy’s National Laboratories. Through the initiative, our Nation’s world-class supercomputers are helping manufacturers achieve significant energy and cost savings, improve product performance, expand their markets, and grow the economy.

High Performance Computing for Manufacturing
Using Supercomputers to improve Energy Efficiency and Performance
To learn more about the HPC4EI program and computational science in general, please download our brochure.
Success Stories
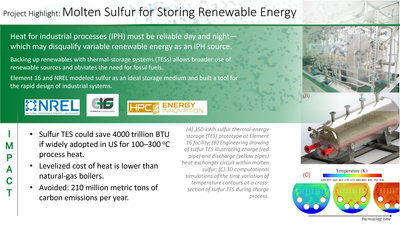
Molten-Sulfur Storage
National Renewable Energy Laboratory and Element 16 Technologies, Inc. partnered through HPC4Mfg to model sulfur as an ideal storage medium and built a tool for the rapid design of industrial systems.
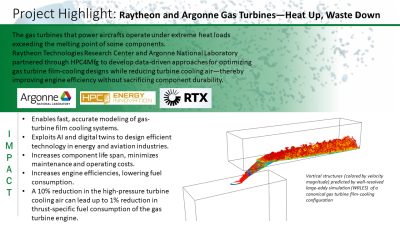
Gas Turbines and HPC
Argonne National Laboratory and Raytheon Technologies Research Center partnered through HPC4Mfg to develop data-driven approaches for optimizing gas turbine film-cooling designs while reducing turbine cooling air—thereby improving engine efficiency without sacrificing component durability.
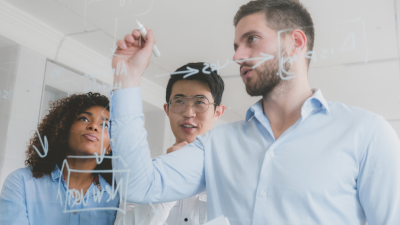
More Success Stories
Check out how the HPC4Mfg Program is contributing to a broad national impact by increasing energy efficiency and advancing energy technologies.
DOE Sponsors
High Performance Computing for Energy Innovation (HPC4EI) is sponsored by U.S. Department of Energy (DOE) Offices:



Partner Labs
Subscribe
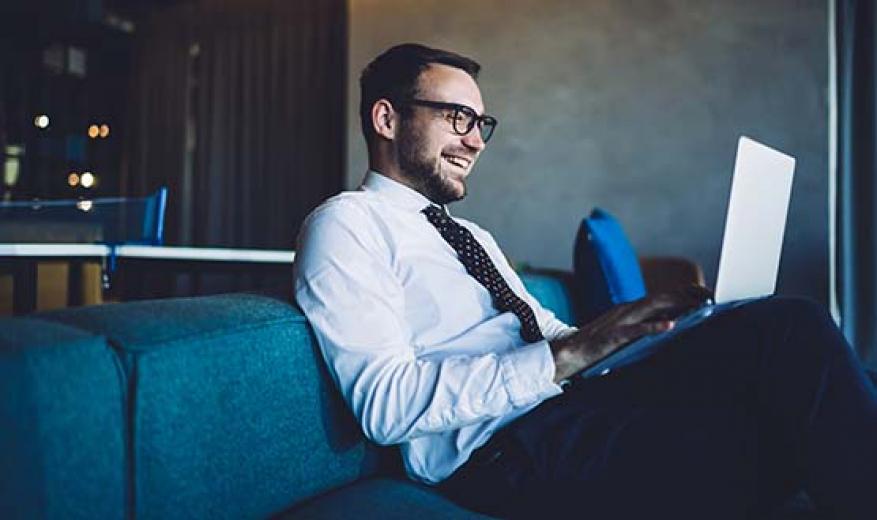