Success Stories
Glass Furnace Model Enhancement

Vitro Glass | Lawerence Livermore National Laboratory
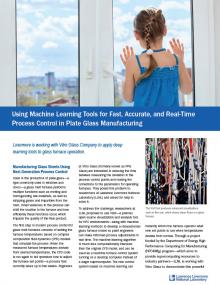
Livermore Lab is working with Vitro Glass Company to provide fast, accurate, and real-time process control in plate glass manufacturing.
Impact
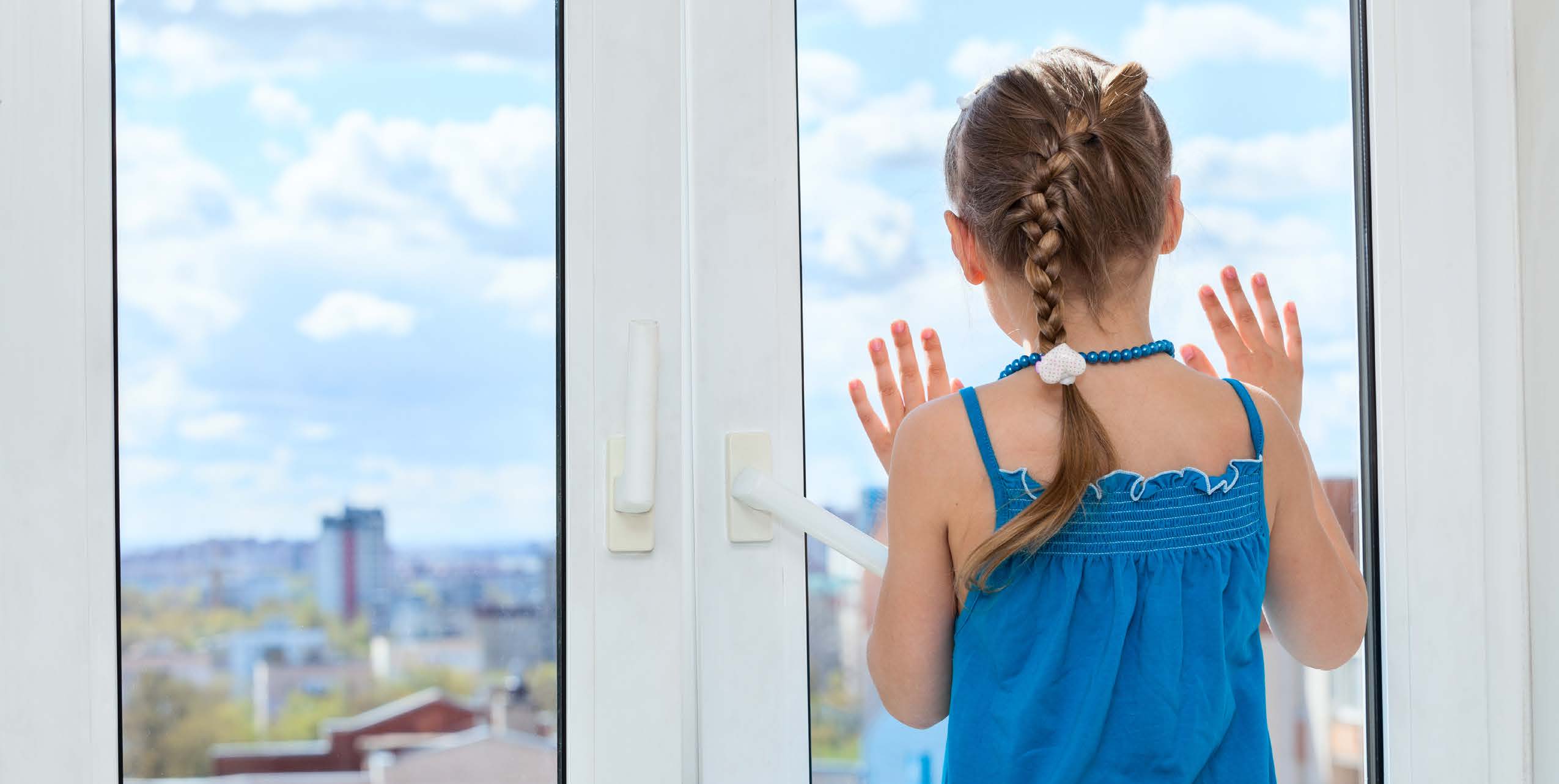
Using Machine Learning Tools for Fast, Accurate, and Real-Time Process Control in Plate Glass Manufacturing
Livermore is working with Vitro Glass Company to apply deep learning tools to glass furnace operation.
Manufacturing Glass Sheets Using Next-Generation Process Control

Used in the production of plate glass—a type commonly used in windows and doors—a glass melt furnace performs multiple functions such as melting and homogenizing raw materials, as well as stripping gases and impurities from the melt. Small variances in the process can shift the location in the furnace and how efficiently these functions occur, which impacts the quality of the final product.
The first step in modern process control for glass melt furnaces consists of setting the furnace temperatures based on complex computation fluid dynamics (CFD) models that simulate the process. When the measured furnace temperatures deviate from desired temperatures, the CFD code is run again to tell operators how to adjust the furnace set points—a process that currently takes up to two weeks. Engineers at Vitro Glass (formerly known as PPG Glass) are interested in reducing the time between measuring the deviation in the process control points and making the corrections to the parameters for operating furnaces. They posed this problem to researchers at Lawrence Livermore National Laboratory (LLNL) and asked for help to solve it.
To address the challenge, researchers at LLNL proposed to use VisIt—a premier, open source visualization and analysis tool for high performance computing (HPC) environments—along with machine learning methods to develop a reduced-order glass furnace model so plant engineers can make informed process adjustments in real time. The machine learning algorithm is much less computationally intensive than the original CFD model, and can be used as a real-time furnace control system running on a desktop computer instead of a larger supercomputer. The new control system based on machine learning can instantly inform the furnace operator what new set points to use when temperatures deviate from normal. Through a project funded by the Department of Energy High Performance Computing for Manufacturing (HPC4Mfg) program— which aims to provide supercomputing resources to industry partners—LLNL is working with Vitro Glass to demonstrate a new process control approach in what has traditionally been an energy-intensive manufacturing sector. This fast-running prediction tool can save roughly two weeks of production per year (per furnace). It also increases productivity by 2%. Extrapolating those improvements to the entire U.S. glass manufacturing industry suggests that 2.5 TBTUs of energy and 130,000 metric tons of carbon dioxide emissions could be saved.
Training the Machine Learning Tool

Vitro engineers use a complex, high-fidelity CFD code to model the processes that occur in the melt furnace. These simulations were computationally intensive and took one to two weeks to run. As a first step to develop a reduced-order model using machine learning techniques, Vitro engineers ran multiple simulations with different possible temperature set points, and then used this data to train machine learning tools that learn the response of the furnace to changes in set points. From the results of these large simulations, researchers could extract the most relevant process variables out of the tens of process variables currently used as control points and train the machine learning tools—noting changes in the output parameters as a function of variations in the input parameters.
The algorithm could thus learn how the system responds to changes in process parameters that an operator may make, or how it responds to changes in sensor readings of the in situ process. This learned response resulted in a reduced- order model that is highly accurate within the bounds of the training parameters and orders of magnitude faster compared to the original CFD model. Ultimately, this tool could be used to set process parameters for desired outputs incorporating continuous learning as new data is collected.
Tools such as the one developed in this project can be used to understand and visualize important variables such as flow field information and in situ glass composition for which limited direct sensing is possible. Vitro plant operators can now use a real-time graphical user interface to adjust process parameters such as furnace temperature and gas flow through the bubblers, enabling them to immediately see the resulting changes in the molten glass flow pattern. This guarantees that the final product will be of high quality.
Other industrial control scenarios for process control are applying this technique. The glass industry is very interested in how new machine learning tools can assist production. Specific applications such as process control help pave the way for meaningful implementation of these new techniques to enhance manufacturing competitiveness.
How to Work with Us
For more information, visit hpc4mfg.org or contact us at hpc4mfg [at] llnl.gov (hpc4mfg[at]llnl[dot]gov).

Your Success Story Awaits
HPC4EI brings together the diverse set of computational skills and supercomputing capabilities of DOE National Laboratories to increase US industry’s energy efficiency and advance competitiveness. Learn about the next opportunity to partner with the superb talent and high performance computing platforms at DOE National Laboratories.
Have questions?
Please email hpc4ei [at] llnl.gov (subject: HPC4EI%20Assistance%20%28Success%20Story%29) (hpc4ei[at]llnl[dot]gov) for further assistance.
Other Success Stories
Title | Company | Laboratory |
---|---|---|
Microwave-assisted Ceramic Processing | Raytheon | Oak Ridge National Laboratory |
Chemical Vapor Infiltration | General Electric | Oak Ridge National Laboratory |
Microscopic Concentration Gradients | Flash Steelworks, Inc. | Oak Ridge National Laboratory |
Molten-Sulfur Storage | Element 16 Technologies, Inc. | National Renewable Energy Laboratory |
Heat Exchangers | Materials Sciences, LLC | Lawrence Livermore National Laboratory |
Gas Turbines and HPC | Raytheon Technologies Research Center | Argonne National Laboratory |
Next Generation Additive Manufacturing | Seurat Technologies | Lawrence Livermore National Laboratory |
Improved Aluminum Ingot Casting | Arconic | Lawrence Livermore National Laboratory & Oak Ridge National Laboratory |
Next-Generation LEDs | SORAA | Lawrence Livermore National Laboratory |
Energy Efficiency in Paper Processing | Agenda 2020 Technology Alliance | Lawrence Livermore National Laboratory & Berkeley National Laboratory |
Reducing Glass Fiber Breakage | PPG Industries | Lawrence Livermore National Laboratory |
Optimizing Lightweight Materials | Lightweight Innovation for Tomorrow (LIFT) | Lawrence Livermore National Laboratory |
Glass Furnace Model Enhancement | Vitro Glass Company |
Lawrence Livermore National Laboratory |
Improve Water Evaporation Processes | Zoom Essence |
Lawrence Livermore National Laboratory |
About HPC4EI
High Performance Computing for Energy Innovation (HPC4EI) is funded by the Department of Energy’s Energy Efficiency and
Renewable Energy’s (EERE) Advanced Materials and Manufacturing Technologies Office (AMMTO), Industrial Efficiency and Decarbonization Office (IEDO) and Office of Fossil Energy and Carbon Management (FECM). The HPC4EI program pairs industry engineers and scientists with national laboratory computational experts to solve difficult production and design problems aiming to reduce national energy consumption. Since its inception 2015, the HPC4EI program has funded over 182 projects with participation by 11 different national laboratories. The world-class computational capabilities at the national laboratories are used to address problems in steel and aluminum manufacture, jet turbine design and manufacture, advanced materials for light weighting and high temperature, high corrosion applications, chemical processing and many more topic areas.